HEWL S-SAD Merging Statistics
Merging statistics are a useful means to assess data quality in crystallography. However, each statistic has inherent shortcomings. For example, R-merge will appear inflated if the multiplicity is high, and the Pearson correlation coefficients used for \(CC_{1/2}\) are very sensitive to outliers.
Most scaling and merging programs output multiple merging statistics to get around these shortcomings. However, one can imagine that it could also be useful to customize certain parameters, such as how many resolution bins are used. Or, perhaps a better statistic will be developed that is worth implementing.
In this notebook, reciprocalspaceship
is used to compute half-dataset correlation coefficients (\(CC_{1/2}\) and \(CC_{anom}\)) for a dataset collected from a tetragonal hen egg-white lysozyme (HEWL) crystal at 6550 eV. These data are unmerged, but were scaled in AIMLESS. They contain sufficient sulfur anomalous signal to determine a solution by the SAD method. This illustrates the use of rs
to implement a merging routine, create a custom analysis, and could be useful as a
template for other exploratory crystallographic data analyses. As an example, we will compare half-dataset correlations computed using both Pearson and Spearman correlation coefficients.
[1]:
%matplotlib inline
import matplotlib.pyplot as plt
import seaborn as sns
sns.set_context("notebook", font_scale=1.3)
import numpy as np
[2]:
import reciprocalspaceship as rs
[3]:
print(rs.__version__)
0.9.9
Load scaled, unmerged data
This data has been scaled in AIMLESS. The data includes the image number and the scaled I and SIGI values.
[4]:
hewl = rs.read_mtz("data/HEWL_unmerged.mtz")
[5]:
hewl.head()
[5]:
BATCH | I | SIGI | PARTIAL | |||
---|---|---|---|---|---|---|
H | K | L | ||||
0 | 0 | 4 | 137 | 696.5212 | 87.83294 | False |
4 | 520 | 710.6812 | 88.107025 | False | ||
4 | 856 | 672.05634 | 87.75671 | False | ||
4 | 1239 | 642.47485 | 87.90302 | False | ||
4 | 2160 | 655.71783 | 87.74394 | False |
[6]:
print(f"Number of observed reflections: {len(hewl)}")
Number of observed reflections: 816804
Merging with Inverse-Variance Weights
Since the input data are unmerged, we will implement the inverse-variance weighting scheme used by AIMLESS to merge the observations. The weighted average is a better estimator of the true mean than the raw average, and this weighting scheme corresponds to the maximum likelihood estimator of the true mean if we assume that the observations are normally-distributed about the true mean.
The merged intensity for each reflection, \(I_h\), can be determined from the observed intensities, \(I_{h,i}\), and error estimates, \(\sigma_{h,i}\), as follows:
\begin{equation} I_h = \frac{\sum_{i}w_{h,i} I_{h,i}}{\sum_{i} w_{h,i}} \end{equation}
where the weight for each observation, \(w_{h,i}\) is given by:
\begin{equation} w_{h,i} = \frac{1}{(\sigma_{h,i})^2} \end{equation}
The updated estimate of the uncertainty, \(\sigma_{h}\), is given by:
\begin{equation} \sigma_{h} = \sqrt{\frac{1}{\sum_{i} w_{h,i}}} \end{equation}
Let’s start by implementing the above equations in a function that will compute the merged \(I_h\) and \(\sigma_h\). We will use the Pandas groupby methods to apply this function across the unique Miller indices in the DataSet
. If we group Friedel pairs together, we will refer to the quantity as IMEAN, and if we keep the Friedel pairs separate we will refer to the quantities as I(+) and I(-).
[7]:
def merge(dataset, anomalous=False):
"""
Merge dataset using inverse-variance weights.
Parameters
----------
dataset : rs.DataSet
DataSet to be merged containing scaled I and SIGI
anomalous : bool
If True, I(+) and I(-) will be reported. If False,
IMEAN will be reported
Returns
-------
rs.DataSet
Merged DataSet object
"""
ds = dataset.hkl_to_asu(anomalous=anomalous)
ds["w"] = ds['SIGI']**-2
ds["wI"] = ds["I"] * ds["w"]
g = ds.groupby(["H", "K", "L"])
result = g[["w", "wI"]].sum()
result["I"] = result["wI"] / result["w"]
result["SIGI"] = np.sqrt(1 / result["w"])
result = result.loc[:, ["I", "SIGI"]]
result.merged = True
if anomalous:
result = result.unstack_anomalous()
return result
Using anomalous=False
, this function can be used to compute IMEAN and SIGIMEAN by including both Friedel pairs:
[8]:
result1 = merge(hewl, anomalous=False)
[9]:
result1.sample(5)
[9]:
I | SIGI | |||
---|---|---|---|---|
H | K | L | ||
23 | 22 | 9 | 1349.8007 | 22.878157 |
37 | 19 | 0 | 260.0996 | 9.075029 |
17 | 11 | 15 | 146.02324 | 2.895325 |
35 | 1 | 14 | 9.629998 | 3.902024 |
15 | 5 | 18 | 7.837115 | 1.039951 |
Using anomalous=True
, this function can be used to compute I(+), SIGI(+), I(-), and SIGI(-) by separating Friedel pairs:
[10]:
result2 = merge(hewl, anomalous=True)
[11]:
result2.sample(5)
[11]:
I(+) | SIGI(+) | SIGI(-) | I(-) | |||
---|---|---|---|---|---|---|
H | K | L | ||||
20 | 4 | 7 | 790.00696 | 13.43943 | 13.45224 | 819.43054 |
21 | 15 | 16 | 51.543583 | 2.840802 | 2.675156 | 50.629883 |
35 | 8 | 12 | 56.64869 | 2.762924 | 2.779431 | 52.87201 |
16 | 16 | 15 | 517.4729 | 11.513449 | 11.513449 | 517.4729 |
33 | 23 | 3 | 101.954094 | 3.753756 | 3.605523 | 89.131714 |
A variant of the above function is implemented in rs.algorithms
, and we will use that implementation in the next section for computing merging statistics. This function computes IMEAN, I(+), I(-), and associated uncertainties for each unique Miller index.
[12]:
result3 = rs.algorithms.merge(hewl)
[13]:
result3.sample(5)
[13]:
IMEAN | SIGIMEAN | I(+) | SIGI(+) | I(-) | SIGI(-) | N(+) | N(-) | |||
---|---|---|---|---|---|---|---|---|---|---|
H | K | L | ||||||||
38 | 10 | 4 | 454.0382 | 11.617793 | 416.8525 | 21.370535 | 469.6386 | 13.841894 | 8 | 20 |
17 | 9 | 15 | 101.308815 | 2.1820927 | 100.45327 | 3.0412343 | 102.21658 | 3.1326878 | 32 | 31 |
38 | 7 | 8 | 4.571434 | 0.9255639 | 4.8481956 | 1.2750458 | 4.2631493 | 1.3457003 | 20 | 20 |
11 | 5 | 5 | 3991.629 | 47.690647 | 4061.3477 | 66.316765 | 3916.9563 | 68.63234 | 60 | 56 |
27 | 18 | 11 | 14.8991 | 1.0327507 | 10.999734 | 1.4392322 | 19.038132 | 1.4828024 | 20 | 20 |
Merging with 2-fold Cross-Validation
To compute correlation coefficients we will repeatedly split our data into half-datasets. We will do this by randomly splitting the data using the image number. These half-datasets will be merged independently and used to determine uncertainties in the correlation coefficients. We will first write a method to randomly split our data, and then we will then write a method that automates the sampling and merging of multiple half-datasets.
[14]:
def sample_halfdatasets(data):
"""Randomly split DataSet into two equal halves by BATCH"""
batch = data.BATCH.unique().to_numpy(dtype=int)
np.random.shuffle(batch)
halfbatch1, halfbatch2 = np.array_split(batch, 2)
half1 = data.loc[data.BATCH.isin(halfbatch1)]
half2 = data.loc[data.BATCH.isin(halfbatch2)]
return half1, half2
[15]:
def merge_dataset(dataset, nsamples):
"""
Merge DataSet using inverse-variance weighting scheme. This represents the
maximum-likelihood estimator of the mean of the observed intensities assuming
they are independent and normally distributed with the same mean.
Sample means across half-datasets can be used to compute the merging statistics CC1/2 and CCanom.
"""
dataset = dataset.copy()
samples = []
for n in range(nsamples):
half1, half2 = sample_halfdatasets(dataset)
mergedhalf1 = rs.algorithms.merge(half1)
mergedhalf2 = rs.algorithms.merge(half2)
result = mergedhalf1.merge(mergedhalf2, left_index=True, right_index=True, suffixes=(1, 2))
result["sample"] = n
samples.append(result)
return rs.concat(samples).sort_index()
Merge HEWL data
We will now merge the HEWL data, repeatedly sampling across half-datasets in order to assess the distribution of correlation coefficients.
[16]:
# This cell takes a few minutes with nsamples=15
merged = merge_dataset(hewl, 15)
[17]:
merged
[17]:
IMEAN1 | SIGIMEAN1 | I(+)1 | SIGI(+)1 | I(-)1 | SIGI(-)1 | N(+)1 | N(-)1 | IMEAN2 | SIGIMEAN2 | I(+)2 | SIGI(+)2 | I(-)2 | SIGI(-)2 | N(+)2 | N(-)2 | sample | |||
---|---|---|---|---|---|---|---|---|---|---|---|---|---|---|---|---|---|---|---|
H | K | L | |||||||||||||||||
0 | 0 | 4 | 657.99817 | 43.882294 | 657.99817 | 43.882294 | 657.99817 | 43.882294 | 4 | 4 | 662.40204 | 25.35386 | 662.40204 | 25.353859 | 662.40204 | 25.353859 | 12 | 12 | 0 |
4 | 645.79663 | 43.884262 | 645.79663 | 43.884266 | 645.79663 | 43.884266 | 4 | 4 | 666.4745 | 25.35348 | 666.4745 | 25.35348 | 666.4745 | 25.35348 | 12 | 12 | 1 | ||
4 | 662.0198 | 25.348951 | 662.0198 | 25.348951 | 662.0198 | 25.348951 | 12 | 12 | 659.13995 | 43.907776 | 659.13995 | 43.907776 | 659.13995 | 43.907776 | 4 | 4 | 2 | ||
4 | 660.4694 | 33.18889 | 660.4694 | 33.18889 | 660.4694 | 33.18889 | 7 | 7 | 661.946 | 29.271538 | 661.946 | 29.271538 | 661.946 | 29.271538 | 9 | 9 | 3 | ||
4 | 666.3023 | 24.359608 | 666.3023 | 24.35961 | 666.3023 | 24.35961 | 13 | 13 | 639.66833 | 50.654987 | 639.66833 | 50.654987 | 639.66833 | 50.654987 | 3 | 3 | 4 | ||
... | ... | ... | ... | ... | ... | ... | ... | ... | ... | ... | ... | ... | ... | ... | ... | ... | ... | ... | ... |
45 | 10 | 2 | 30.170218 | 7.3511124 | 30.170218 | 7.3511124 | NaN | NaN | 1 | 0 | 15.318142 | 3.9687188 | 15.318142 | 3.9687188 | NaN | NaN | 3 | 0 | 9 |
2 | 7.669444 | 6.6297607 | 7.669444 | 6.6297607 | NaN | NaN | 1 | 0 | 22.89468 | 4.1084785 | 22.89468 | 4.1084785 | NaN | NaN | 3 | 0 | 10 | ||
2 | 11.665072 | 5.2794247 | 11.665072 | 5.2794247 | NaN | NaN | 2 | 0 | 24.119883 | 4.656634 | 24.119883 | 4.656634 | NaN | NaN | 2 | 0 | 11 | ||
2 | 7.669444 | 6.6297607 | 7.669444 | 6.6297607 | NaN | NaN | 1 | 0 | 22.89468 | 4.1084785 | 22.89468 | 4.1084785 | NaN | NaN | 3 | 0 | 12 | ||
2 | 17.961912 | 4.288131 | 17.961912 | 4.288131 | NaN | NaN | 3 | 0 | 20.064919 | 6.0180674 | 20.064919 | 6.0180674 | NaN | NaN | 1 | 0 | 13 |
187364 rows × 17 columns
Compute \(CC_{1/2}\) and \(CC_{anom}\)
We will first assign each reflection to a resolution bin and then we will compute the correlation coefficients
[18]:
merged, labels = merged.assign_resolution_bins(bins=15)
[19]:
groupby1 = merged.groupby(["sample", "bin"])[["IMEAN1", "IMEAN2"]]
pearson1 = groupby1.corr(method="pearson").unstack().loc[:, ("IMEAN1", "IMEAN2")]
pearson1.name = "Pearson"
spearman1 = groupby1.corr(method="spearman").unstack().loc[:, ("IMEAN1", "IMEAN2")]
spearman1.name = "Spearman"
results1 = rs.concat([pearson1, spearman1], axis=1)
results1 = results1.groupby("bin").agg(["mean", "std"])
[20]:
results1.head()
[20]:
Pearson | Spearman | |||
---|---|---|---|---|
mean | std | mean | std | |
bin | ||||
0 | 0.997334 | 0.000316 | 0.998895 | 0.000063 |
1 | 0.997958 | 0.000360 | 0.999363 | 0.000066 |
2 | 0.998971 | 0.000306 | 0.999678 | 0.000023 |
3 | 0.999297 | 0.000102 | 0.999658 | 0.000024 |
4 | 0.999368 | 0.000147 | 0.999687 | 0.000022 |
[21]:
plt.figure(figsize=(8, 4))
plt.errorbar(results1.index, results1[("Pearson", "mean")],
yerr=results1[("Pearson", "std")],
color="#1b9e77",
label=r"$CC_{1/2}$ (Pearson)")
plt.errorbar(results1.index, results1[("Spearman", "mean")],
yerr=results1[("Spearman", "std")],
color="#d95f02",
label=r"$CC_{1/2}$ (Spearman)")
plt.xticks(results1.index, labels, rotation=45, ha='right', rotation_mode='anchor')
plt.ylabel("Correlation Coefficient")
plt.xlabel(r"Resolution Bin ($\AA$)")
plt.legend(loc='center left', bbox_to_anchor=(1, 0.5))
plt.grid(axis="y", linestyle='--')
plt.show()
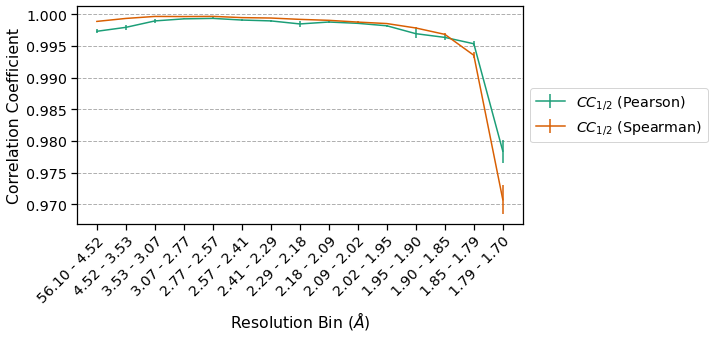
It is important to note the scale on the y-axis – this dataset is edge-limited, and as such the \(CC_{1/2}\) is very high across all resolution bins. The Spearman CC appears higher across all resolution bins except at high resolution, and overall has a lower standard deviation among samples. This is consistent with our expectation that Spearman CCs are a more robust estimator of correlation than Pearson CCs.
Let’s now repeat this for the anomalous data, computing \(CC_{anom}\):
[22]:
merged["ANOM1"] = merged["I(+)1"] - merged["I(-)1"]
merged["ANOM2"] = merged["I(+)2"] - merged["I(-)2"]
# Similar to CChalf, but we will only look at acentric reflections
groupby2 = merged.acentrics.groupby(["sample", "bin"])[["ANOM1", "ANOM2"]]
pearson2 = groupby2.corr(method="pearson").unstack().loc[:, ("ANOM1", "ANOM2")]
pearson2.name = "Pearson"
spearman2 = groupby2.corr(method="spearman").unstack().loc[:, ("ANOM1", "ANOM2")]
spearman2.name = "Spearman"
results2 = rs.concat([pearson2, spearman2], axis=1)
results2 = results2.groupby("bin").agg(["mean", "std"])
[23]:
results2.head()
[23]:
Pearson | Spearman | |||
---|---|---|---|---|
mean | std | mean | std | |
bin | ||||
0 | 0.422196 | 0.037998 | 0.497923 | 0.029704 |
1 | 0.233349 | 0.061975 | 0.328263 | 0.023917 |
2 | 0.357182 | 0.051746 | 0.444657 | 0.029250 |
3 | 0.484710 | 0.045454 | 0.543314 | 0.031731 |
4 | 0.579058 | 0.023952 | 0.611739 | 0.020141 |
[24]:
plt.figure(figsize=(8, 4))
plt.errorbar(results2.index, results2[("Pearson", "mean")],
yerr=results2[("Pearson", "std")],
color="#1b9e77",
label=r"$CC_{anom}$ (Pearson)")
plt.errorbar(results2.index, results2[("Spearman", "mean")],
yerr=results2[("Spearman", "std")],
color="#d95f02",
label=r"$CC_{anom}$ (Spearman)")
plt.xticks(results2.index, labels, rotation=45, ha='right', rotation_mode='anchor')
plt.ylabel("Correlation Coefficient")
plt.xlabel(r"Resolution Bin ($\AA$)")
plt.legend(loc='center left', bbox_to_anchor=(1, 0.5))
plt.grid(axis="y", linestyle='--')
plt.show()
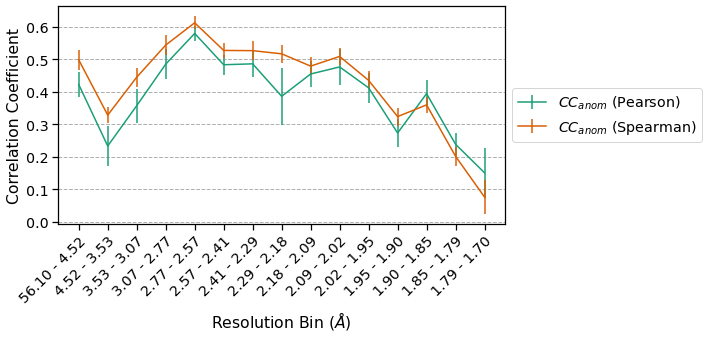
There is significant anomalous signal across all but the highest resolution bins. The Spearman CCs have smaller error bars than the corresponding Pearson CCs and the Spearman CCs are also higher in most bins. These differences highlight the influence of outlier measurements on the different correlation coefficients.
Summary
We have used reciprocalspaceship
to merge a dataset using inverse-variance weights. As part of this analysis, we performed repeated 2-fold cross-validation to compute Pearson and Spearman correlation coefficients and associated uncertainties. This relatively simple procedure to obtain uncertainty estimates for correlation coefficients is seldom done when analyzing merging quality. However, we can see that the standard deviation for computed correlation coefficients can be nearly
\(\pm0.1\) for quantities such as \(CC_{anom}\). This is worth keeping in mind when analyzing SAD experiments because this dataset is very high quality (edge-limited) when one considers the \(CC_{1/2}\). Putting these two correlation coefficients on the same axes emphasizes this point:
[25]:
plt.figure(figsize=(9, 6))
plt.errorbar(results1.index, results1[("Pearson", "mean")],
yerr=results1[("Pearson", "std")],
color='#1b9e77',
label=r"$CC_{1/2}$ (Pearson)")
plt.errorbar(results1.index, results1[("Spearman", "mean")],
yerr=results1[("Spearman", "std")],
color='#d95f02',
label=r"$CC_{1/2}$ (Spearman)")
plt.errorbar(results2.index, results2[("Pearson", "mean")],
yerr=results2[("Pearson", "std")],
color='#1b9e77',
linestyle="--",
label=r"$CC_{anom}$ (Pearson)")
plt.errorbar(results2.index, results2[("Spearman", "mean")],
yerr=results2[("Spearman", "std")],
color='#d95f02',
linestyle="--",
label=r"$CC_{anom}$ (Spearman)")
plt.xticks(results1.index, labels, rotation=45, ha='right', rotation_mode='anchor')
plt.ylabel(r"Correlation Coefficient")
plt.xlabel(r"Resolution Bin ($\AA$)")
plt.legend(loc='center left', bbox_to_anchor=(1, 0.5))
plt.grid(axis="y", linestyle='--')
plt.tight_layout()
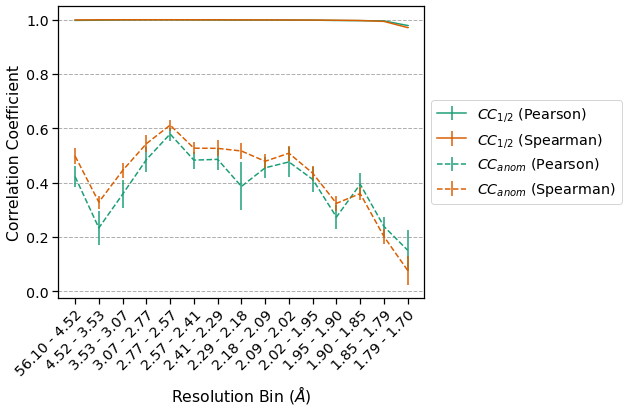
Even a simple change to the procedure for computing merging statistics, such as substituting a Spearman correlation coefficient for a Pearson one, can alter the apparent quality of a dataset. By lowering the barrier to implementing new analyses, we hope that reciprocalspaceship
can encourage the development of more robust indicators of crystallographic data quality.